The digital transformation of supply chain management is increasingly vital in the current competitive business environment. A pivotal innovation in this realm is the concept of Digital Twins, which are virtual replicas of physical assets, processes, or systems. This article explores how Digital Twins can be harnessed to simulate and optimise Demand-Driven Material Requirements Planning (DDMRP) processes, enhancing efficiency, inventory management, and predictive maintenance capabilities.
Understanding DDMRP
Demand-Driven Material Requirements Planning (DDMRP) is a methodology that integrates traditional MRP (Material Requirements Planning) and DRP (Distribution Requirements Planning) with lean and Six Sigma principles. It focuses on adjusting inventory levels based on actual demand, rather than forecasts, to improve responsiveness and reduce stockouts and excess inventory.
Core Components of DDMRP
- Strategic Inventory Positioning: This involves pinpointing critical points within the supply chain where inventory should be placed to decouple dependencies and ensure smooth material flow. By strategically positioning inventory, companies can create buffers to absorb variability in both supply and demand, preventing disruptions in one part of the supply chain from impacting the entire system.
- Buffer Profiles and Levels: Buffers are crucial to DDMRP, and establishing the right buffer profiles and levels is key for effective supply chain management. Buffer profiles are set based on factors like lead times, variability, and demand patterns. Buffer levels are dynamically adjusted to maintain optimal inventory, avoiding both stockouts and overstocking. These buffers are divided into three zones: green (replenishment quantity), yellow (lead time stock), and red (safety quantity).
- Dynamic Adjustments: Dynamic adjustments involve continuously updating buffer levels and positions based on real-time data and changing conditions. This process includes regularly reviewing and tweaking buffer levels to match actual demand and supply situations. By dynamically adjusting buffers, companies can maintain an optimal balance between inventory levels and service levels, reducing excess inventory and enhancing responsiveness.
- Demand-Driven Planning: This approach shifts the focus from forecast-driven to actual demand-driven operations. In this method, replenishment and production decisions are based on real-time demand signals rather than forecasts. This improves supply chain responsiveness, allowing companies to quickly adapt to changes in demand and minimise the risk of overproduction or stockouts.
- Visible and Collaborative Execution: Emphasises transparency and cooperation across the supply chain by sharing real-time data and insights among stakeholders, enabling quick responses to changes and informed decision-making.
- Tactical Adaptation: Tactical adaptation involves managing the evolution of DDMRP through Demand Driven Sales and Operations Planning (DDS&OP). This process adjusts the model based on past performance and anticipated future activities, boosting overall effectiveness and eliminating the need for traditional master production schedules (MPS).
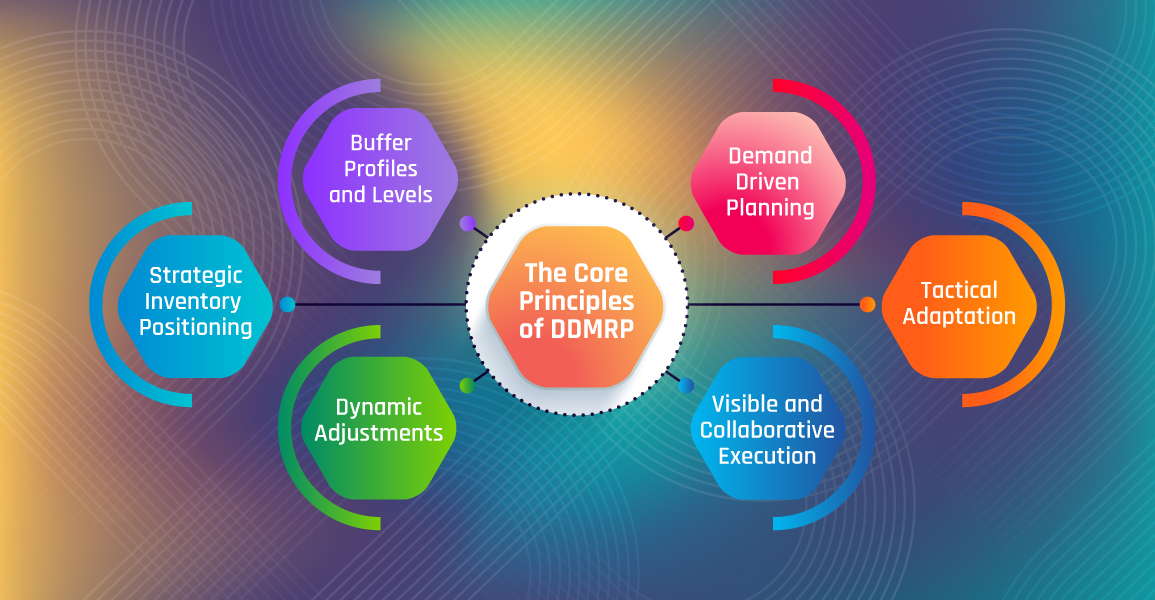
Digital Twins: An Overview
A Digital Twin is a dynamic digital representation of a physical object, process, or system that mirrors its real-world counterpart in real-time. It leverages data from sensors and other sources to create a comprehensive model that evolves with the physical system. This digital model can simulate various scenarios, analyse performance, and predict outcomes, providing valuable insights for decision-making.
Key Features of Digital Twins
Real-Time Data Integration: One of the primary features of Digital Twins is real-time data integration. This capability involves the continuous collection and assimilation of data from multiple sources such as sensors, enterprise systems, and external environments. By maintaining an up-to-date digital representation, organisations can monitor the current state of their systems with high accuracy. This real-time visibility allows for immediate detection of anomalies, quicker response times, and more informed decision-making. For instance, in a manufacturing setting, real-time data from machinery can be used to monitor performance and detect deviations from normal operating conditions, thereby preventing potential breakdowns and minimising downtime.
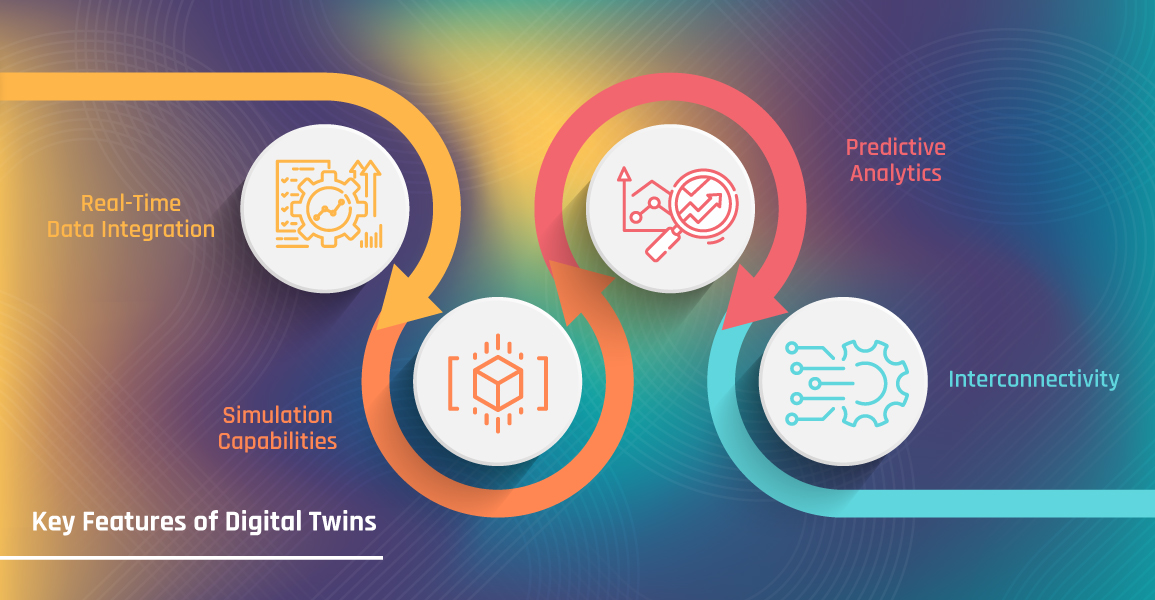
Simulation Capabilities: Digital Twins excel in running detailed simulations, which are crucial for predicting outcomes and testing various scenarios without impacting the actual system. These simulations can model different operational conditions, stress test processes, and evaluate the effects of potential changes. This feature is particularly beneficial for optimising processes and identifying the best course of action before implementation. For example, in supply chain management, a Digital Twin can simulate the impact of changes in demand, supply chain disruptions, or new process implementations, helping managers to devise optimal strategies and avoid costly mistakes.
Predictive Analytics: Predictive analytics is another standout feature of Digital Twins. By leveraging advanced algorithms and machine learning techniques, Digital Twins can analyse historical and real-time data to forecast future states and identify potential issues before they arise. This capability is invaluable for proactive maintenance, demand forecasting, and risk management. For instance, in the context of predictive maintenance, Digital Twins can predict when a piece of equipment is likely to fail based on patterns in the data, allowing for timely maintenance interventions that prevent unexpected downtime and extend the equipment’s life cycle.
Interconnectivity: Interconnectivity is a defining characteristic of Digital Twins, enabling seamless communication between the digital and physical worlds. This feature allows for real-time updates and interactions, where changes in the physical system are instantly reflected in the digital model and vice versa. This interconnectedness facilitates synchronised operations, automated adjustments, and remote management. In industries like energy management, Digital Twins can remotely control and optimise energy distribution networks, ensuring efficient operation and rapid response to changes in demand or supply conditions.
The Synergy Between Digital Twins and DDMRP
The integration of Digital Twins into DDMRP processes offers significant advantages by enabling detailed simulation, analysis, and optimization. This synergy can transform supply chain management, leading to improved efficiency, reduced costs, and enhanced responsiveness.
Enhanced Supply Chain Visibility
Digital Twins offer a comprehensive view of the entire supply chain, enabling real-time monitoring and analysis. This enhanced visibility allows organisations to identify bottlenecks, inefficiencies, and potential disruptions early, facilitating proactive decision-making.
- Real-Time Monitoring: Continuous data collection and integration from various points in the supply chain enable real-time monitoring of inventory levels, production rates, and transportation status. This immediate visibility ensures that any deviations from the norm are quickly detected and addressed.
- Predictive Insights: By leveraging advanced analytics, Digital Twins can forecast demand trends, potential stockouts, and production delays. These predictive insights allow organisations to intervene timely, adjusting strategies to mitigate risks and capitalise on opportunities.
- Scenario Analysis: Digital Twins can simulate various scenarios, such as changes in demand, supplier delays, or production issues. This capability helps evaluate their impact on the supply chain and identify optimal strategies for different situations. By testing potential changes in a virtual environment, companies can make more informed decisions, enhancing overall supply chain resilience and efficiency.
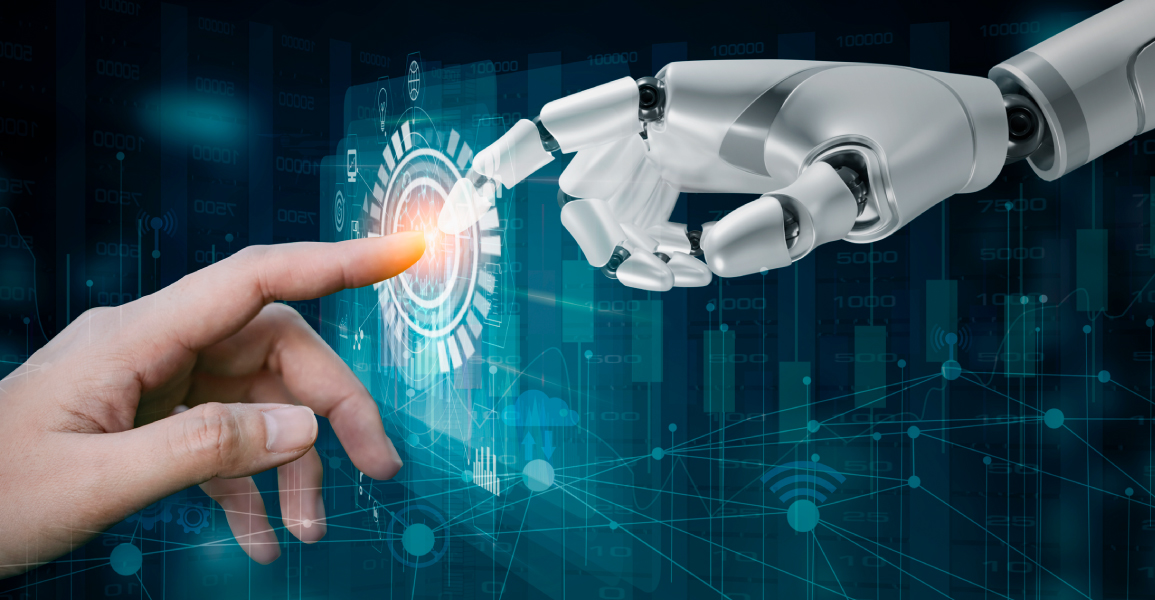
Optimization of Inventory Management
Inventory management is a critical aspect of Demand-Driven Material Requirements Planning (DDMRP), and Digital Twins can significantly enhance this process by optimising buffer levels and reducing carrying costs.
- Dynamic Buffer Adjustments: Digital Twins continuously analyse demand patterns and supply variability to dynamically adjust buffer levels, ensuring optimal inventory positions. This real-time adaptability helps maintain the right amount of inventory, avoiding both shortages and excess.
- Cost Reduction: By optimising inventory levels, Digital Twins help reduce carrying costs associated with excess inventory and minimise stock outs that can lead to lost sales. This efficient inventory management ensures that resources are used effectively, leading to substantial cost savings.
- Performance Metrics: Detailed performance metrics and key performance indicators (KPIs) can be tracked and analysed through Digital Twins to identify areas for improvement. This data-driven approach ensures that inventory management practices align with organisational goals and performance standards. Continuous monitoring and analysis enable companies to refine their strategies and maintain high levels of efficiency.
Facilitating Predictive Maintenance
Predictive maintenance is another area where Digital Twins can add substantial value. By predicting equipment failures and maintenance needs, organisations can avoid costly downtime and ensure smooth operations.
- Monitoring: Real-time data from sensors continuously monitors the condition of equipment, identifying signs of wear and potential failures before they occur. This proactive approach enables timely interventions, preventing unexpected breakdowns and maintaining operational efficiency.
- Maintenance Scheduling: Predictive analytics, powered by Digital Twins, determine the optimal time for maintenance. This balances the need for reliability with minimising disruptions to production. By scheduling maintenance activities at the most opportune moments, organisations can ensure that equipment remains in optimal condition without adversely affecting productivity.
- Cost Savings: Predictive maintenance significantly reduces unplanned downtime and extends the lifespan of equipment, leading to substantial cost savings over time. By preventing sudden equipment failures and optimising maintenance schedules, organisations can avoid the high costs associated with emergency repairs and lost production time.
Implementing Digital Twins in DDMRP Processes
The implementation of Digital Twins in DDMRP processes involves several steps, including data integration, model development, and continuous monitoring and optimization.
Data Integration
The foundation of a successful Digital Twin implementation is the integration of real-time data from various sources within the supply chain.
- Sensor Integration: Deploying sensors at critical points in the supply chain to collect real-time data on inventory levels, production rates, and equipment conditions.
- Data Aggregation: Aggregating data from different sources into a centralised system for comprehensive analysis and decision-making.
- Data Quality: Ensuring high-quality data by implementing robust data governance practices and leveraging advanced data cleansing and validation techniques.
Model Development
Developing an accurate and dynamic digital model of the DDMRP process is crucial for effective simulation and optimization.
- System Mapping: Creating a detailed map of the entire supply chain, including inventory positions, production processes, and transportation networks.
- Model Calibration: Calibrating the digital model using historical data and real-time inputs to ensure its accuracy and reliability.
- Continuous Improvement: Continuously refining the model based on feedback and new data to enhance its predictive capabilities and ensure alignment with real-world conditions.
Monitoring and Optimization
Once the Digital Twin is developed, continuous monitoring and optimization are essential to maximise its benefits.
- Real-Time Dashboards: Implementing real-time dashboards that provide visibility into key performance metrics and alert users to potential issues.
- Automated Decision-Making: Leveraging advanced analytics and machine learning algorithms to automate decision-making processes, such as dynamic buffer adjustments and maintenance scheduling.
- Continuous Feedback Loop: Establishing a feedback loop where insights from the Digital Twin are used to make improvements in the physical system, which in turn enhances the accuracy and effectiveness of the digital model.
Future Trends and Innovations
The integration of Digital Twins and DDMRP processes is expected to evolve further, driven by advancements in technology and increasing adoption across industries.
AI and Machine Learning
Artificial intelligence (AI) and machine learning (ML) are poised to play a significant role in enhancing the capabilities of Digital Twins.
- Predictive Analytics: AI and ML algorithms can improve the accuracy of predictive analytics, enabling more precise forecasts of demand, inventory needs, and equipment failures.
- Automated Optimization: Machine learning can automate the optimization of buffer levels, production schedules, and maintenance plans, reducing the need for manual intervention.
- Anomaly Detection: AI can enhance the ability to detect anomalies and potential issues in the supply chain, enabling proactive measures to prevent disruptions.
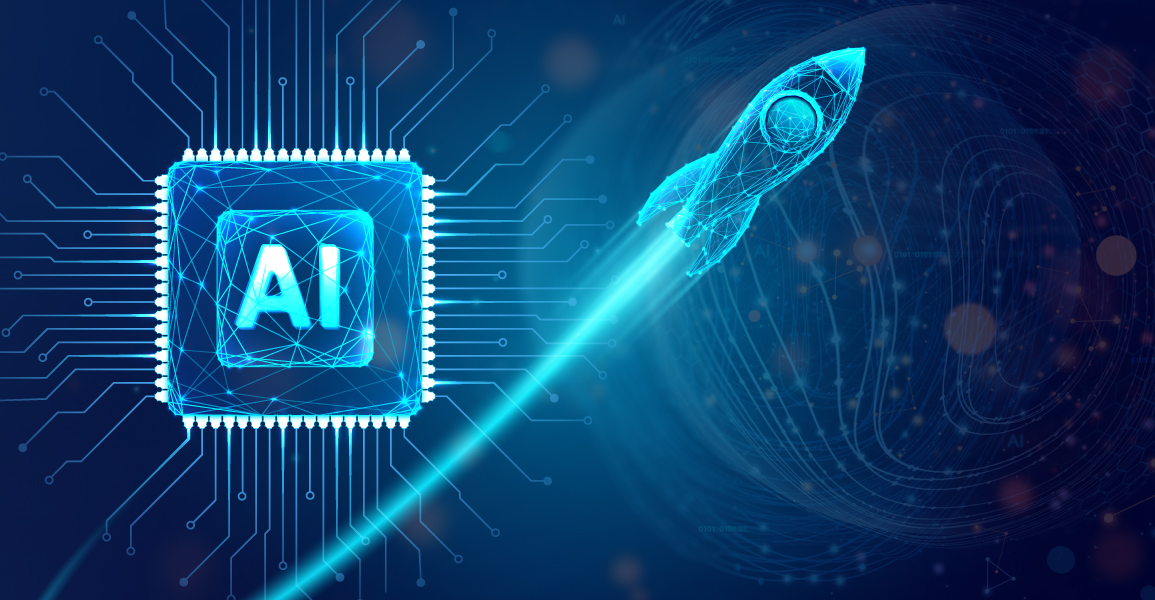
IoT and Edge Computing
The Internet of Things (IoT) and edge computing are expected to further enhance the real-time capabilities of Digital Twins.
- Sensor Integration: The proliferation of IoT devices will enable more comprehensive data collection from various points in the supply chain.
- Edge Processing: Edge computing will allow for real-time data processing and analysis at the source, reducing latency and enabling faster decision-making.
- Scalability: The combination of IoT and edge computing will enable the scalability of Digital Twin implementations across large and complex supply chains.
Conclusion
The integration of Digital Twins in simulating and optimising DDMRP processes represents a significant advancement in supply chain management. By providing enhanced visibility, optimising inventory management, and facilitating predictive maintenance, Digital Twins can transform the way organisations manage their supply chains. As technology continues to evolve, the synergy between Digital Twins and DDMRP will unlock new opportunities for efficiency, cost savings, and responsiveness in supply chain operations.
Organisations that embrace this innovation will be better positioned to navigate the complexities of the modern supply chain landscape and achieve sustainable competitive advantages.
Explore how digital twins can revolutionise your DDMRP strategy. Get in touch today!
