In today’s volatile market landscape, traditional supply chain management approaches often fall short in addressing the complexities and rapid shifts in demand. Dynamic Demand Management (DDM) emerges as a strategic approach that enhances the flexibility and responsiveness of supply chains. At the heart of DDM lies the Demand Driven Material Requirements Planning (DDMRP) framework, which integrates elements of lean manufacturing, theory of constraints, and six sigma methodologies to optimise inventory levels and improve service performance.
This article delves into the concept of dynamic demand management within the DDMRP framework, exploring techniques for adjusting DDMRP parameters such as buffer sizing, order policies, and lead times in response to fluctuating market conditions. It also discusses the pivotal role of data analytics, predictive modelling, and demand sensing technologies in optimising DDMRP for maximum agility and resilience.
Understanding DDMRP: A Brief Overview
Demand Driven Material Requirements Planning (DDMRP) is an innovative supply chain management methodology designed to enhance the flexibility and responsiveness of inventory systems. It combines elements of traditional Material Requirements Planning (MRP), Lean Manufacturing, and the Theory of Constraints to create a dynamic and demand driven approach to managing materials and production.
The Core Principles of DDMRP
DDMRP is structured around six core components:
- Strategic Inventory Positioning: Focuses on placing inventory buffers strategically within the supply chain to decouple lead times, mitigate variability, and stabilise production and delivery processes.
- Buffer Profiles and Levels: Manages inventory dynamically with green, yellow, and red zones indicating target, warning, and critical replenishment levels. Buffer sizes are adjusted based on lead time, demand variability, and order frequency to maintain optimal inventory.
- Dynamic Adjustments: Involves continuous recalibration of buffer sizes and parameters in response to real-time data and market changes, ensuring flexibility and responsiveness.
- Demand Driven Planning: Shifts focus from forecast-driven to sales-driven replenishment, using real-time demand signals to align inventory with actual sales orders, reducing lead times and improving service levels.
- Visible and Collaborative Execution: Emphasises transparency and cooperation across the supply chain by sharing real-time data and insights among stakeholders, enabling quick responses to changes and informed decision-making.
- Tactical Adaptation: Involves managing the adaptation of DDMRP through Demand Driven Sales and Operations Planning (DDS&OP), which adjusts the model based on past performance and anticipated future activities, enhancing overall effectiveness and eliminating the need for traditional master production schedules (MPS).
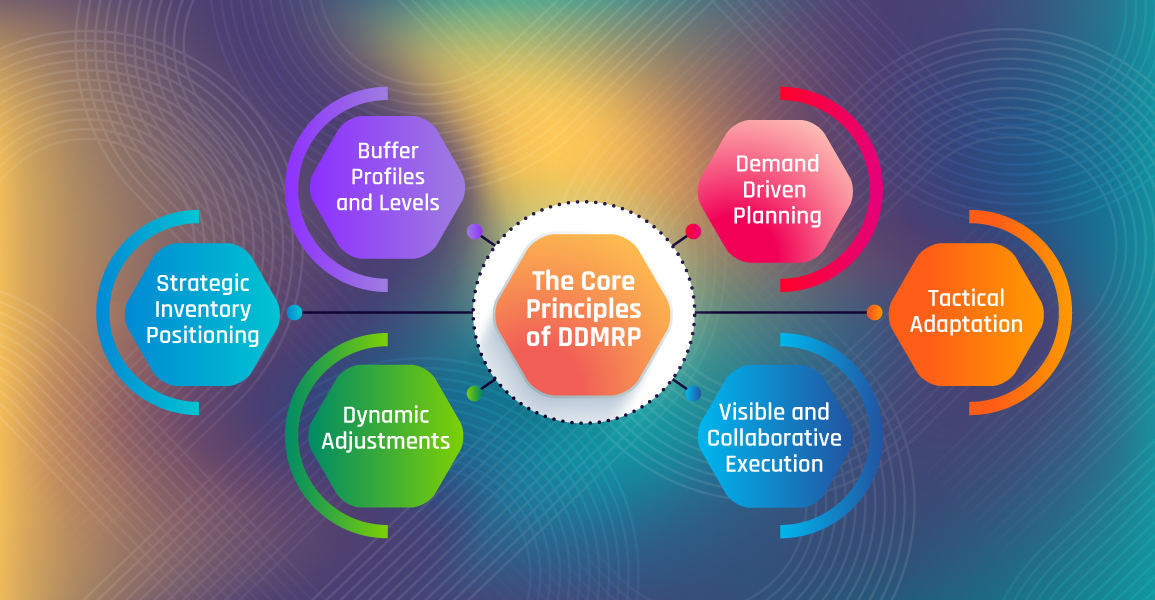
The Need for Dynamic Demand Management
Traditional planning systems rely heavily on historical data and static forecasts, which often fail to account for sudden market shifts. This can lead to overstocking, stockouts, and inefficient resource allocation. Dynamic Demand Management addresses these issues by leveraging real-time data and adaptive strategies to maintain optimal inventory levels and meet customer demand effectively.
Adjusting DDMRP Parameters for Market Dynamics
Buffer Sizing: Adapting to Demand Variability
Buffer sizing is a critical aspect of Demand Driven Material Requirements Planning (DDMRP), designed to absorb fluctuations in demand and supply. Effective buffer sizing helps maintain optimal inventory levels, ensuring that supply chains remain resilient and responsive to market changes. The size of the buffer is influenced by several key factors, including lead time, order frequency, and demand variability.
- Real-Time Data Integration: Incorporating real-time sales and inventory data is essential for continuously recalibrating buffer sizes. By leveraging advanced data analytics and demand sensing technologies, businesses can monitor current market conditions and adjust buffer zones—green (optimal), yellow (caution), and red (critical)—accordingly. This real-time integration ensures that inventory levels are always aligned with actual demand, reducing the risk of stockouts and overstock situations. Continuous data updates enable dynamic adjustments, making the supply chain more agile and responsive.
- Seasonal Adjustments: Demand variability often follows predictable patterns, such as seasonal fluctuations or promotional events. During peak seasons, buffer sizes can be expanded to prevent stockouts and ensure that customer demand is met promptly. This proactive approach helps maintain service levels during high-demand periods. Conversely, during periods of low demand, buffers can be reduced to minimise carrying costs and avoid excess inventory. Seasonal adjustments are critical for optimising inventory levels throughout the year, balancing the need for availability with cost efficiency.
- Lead Time Compression: Lead time compression involves reducing the time required to replenish inventory through improved supplier collaboration and faster logistics. Shorter lead times decrease the required buffer size, leading to more efficient inventory management. By working closely with suppliers to streamline production and delivery processes, businesses can achieve more predictable and reliable lead times. This reduction in lead time variability allows for smaller, more precise buffers, which in turn reduces carrying costs and enhances overall supply chain efficiency.
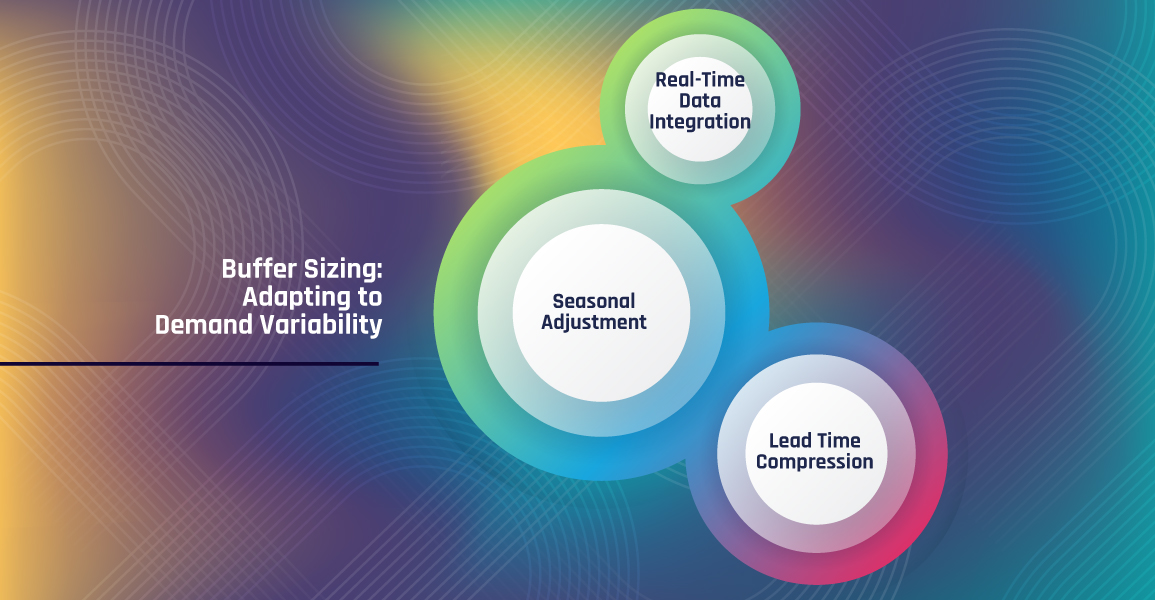
Order Policies: Balancing Supply and Demand
Order policies are crucial in DDMRP as they dictate how and when replenishment orders are triggered, ensuring that supply aligns with fluctuating demand. Dynamic adjustment of these policies is essential for maintaining optimal inventory levels and preventing both stockouts and excess stock.
- Adaptive Order Frequencies: Adaptive order frequencies are a key component of effective order policies. During high-demand periods, increasing order frequencies helps maintain inventory levels without leading to overstocking. Conversely, during low-demand periods, reducing order frequencies can minimise unnecessary inventory buildup, thereby lowering carrying costs and enhancing overall efficiency.
- Lot Size Optimisation: Optimising lot sizes based on current demand and lead time variations ensures that replenishments are both cost-effective and timely. By adjusting the size of each order to reflect real-time demand conditions, businesses can reduce holding costs and improve inventory turnover rates. This tailored approach allows for more precise inventory management and better alignment with market needs.
- Emergency Replenishment Protocols: Implementing emergency replenishment protocols for critical items is essential during unexpected demand spikes. These protocols enable quick responses to sudden increases in demand, preventing stockouts and ensuring that service levels are maintained. By having contingency plans in place, businesses can safeguard against supply chain disruptions and maintain customer satisfaction even during unforeseen events.
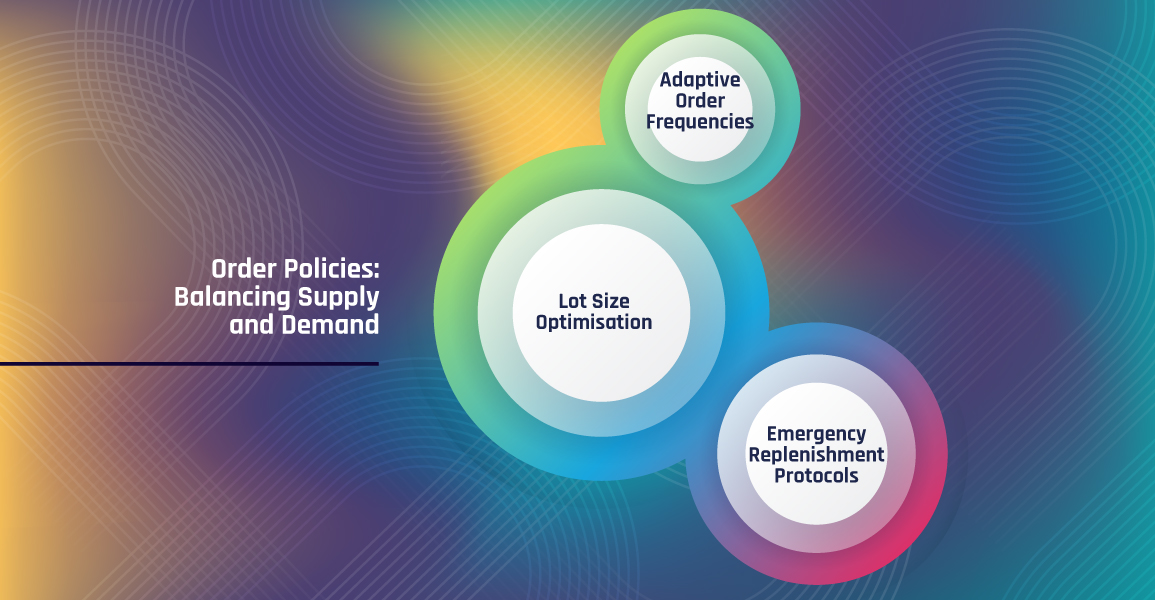
Lead Times: Enhancing Supply Chain Agility
Lead time management is crucial for maintaining supply chain agility and responsiveness. Reducing and stabilising lead times can significantly improve the effectiveness of Demand Driven Material Requirements Planning (DDMRP).
- Supplier Collaboration: Building strong relationships with suppliers and leveraging their capabilities are essential for reducing lead times and ensuring more reliable deliveries. Close collaboration fosters better communication, enabling suppliers to anticipate needs and respond more quickly to demand changes. This partnership approach can streamline production schedules and reduce delays, resulting in shorter and more predictable lead times.
- Flexible Logistics Solutions: Employing flexible logistics strategies, such as multi-modal transportation and decentralised warehousing, enhances lead time predictability and reduces variability. Multi-modal transportation allows businesses to choose the most efficient combination of shipping methods, optimising cost and speed. Decentralised warehousing places inventory closer to end customers, shortening delivery times and increasing responsiveness. These flexible solutions enable quicker adjustments to changing market conditions and demand fluctuations.
- Technology Integration: Utilising advanced technologies like the Internet of Things (IoT) and blockchain for real-time tracking and transparency significantly improves lead time management. IoT devices provide real-time data on inventory levels, shipment locations, and environmental conditions, allowing for proactive adjustments and rapid responses to issues. Blockchain technology ensures secure and transparent tracking of goods throughout the supply chain, reducing the risk of delays and enhancing trust among stakeholders. These technologies facilitate more accurate and reliable lead time management, contributing to a more agile and responsive supply chain.
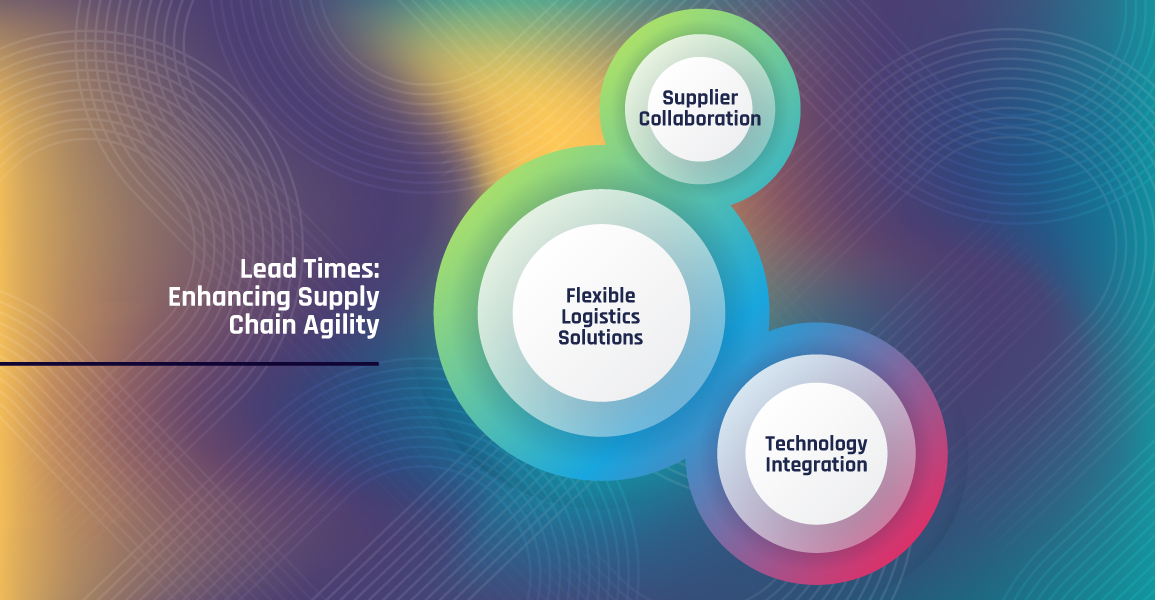
Leveraging Data Analytics and Predictive Modeling
The Role of Data Analytics in DDMRP
Data analytics plays a pivotal role in optimising DDMRP by providing insights into demand patterns, inventory levels, and supply chain performance.
- Descriptive Analytics: Descriptive analytics involves analysing historical data to understand past performance and identify trends and anomalies in demand and supply. This foundational step helps businesses establish a baseline understanding of their supply chain dynamics, revealing patterns and highlighting areas that may require attention. By comprehensively reviewing past data, companies can better prepare for recurring trends and manage deviations effectively.
- Diagnostic Analytics: Diagnostic analytics takes a step further by investigating the root causes of variances in demand and supply. This type of analysis helps identify specific factors contributing to performance issues or unexpected changes. By pinpointing these root causes, businesses can implement targeted corrective actions and adjust their DDMRP policies to mitigate similar issues in the future. Diagnostic analytics thus provides a crucial link between data observation and actionable insights.
- Prescriptive Analytics: Prescriptive analytics employs optimization algorithms to recommend the best course of action for inventory management, order policies, and buffer adjustments. This advanced form of analytics not only predicts future scenarios but also suggests optimal strategies to enhance supply chain efficiency. By leveraging prescriptive analytics, businesses can make data-driven decisions that align inventory levels with actual demand, ensuring that buffers are appropriately sized and orders are timed to meet market needs precisely.
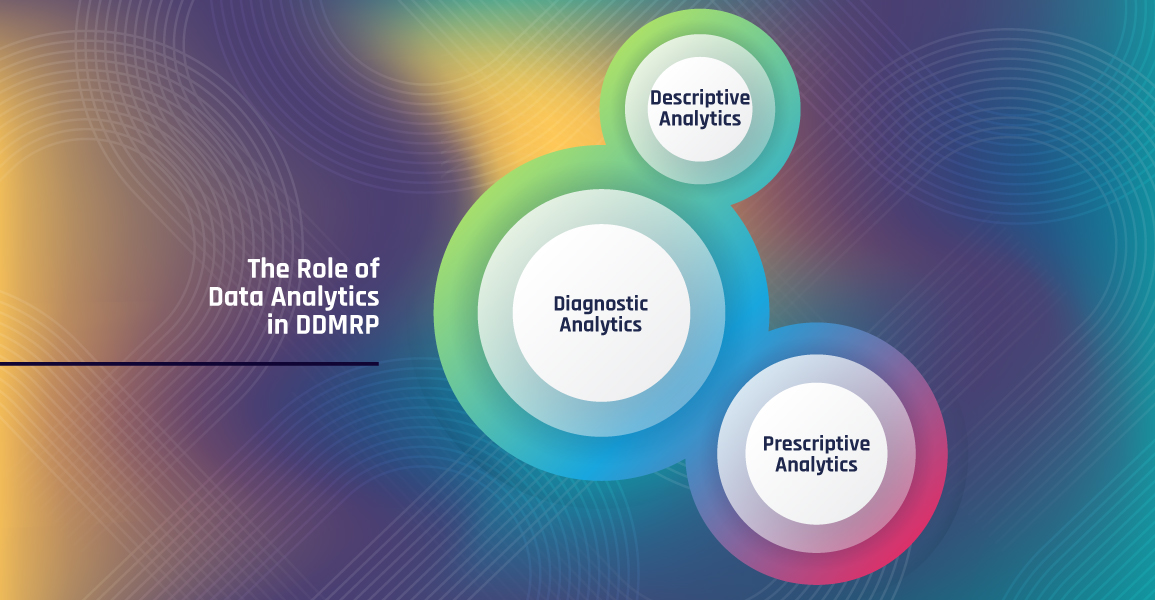
Predictive Modelling for Demand Forecasting
Predictive modelling involves using statistical and machine learning techniques to forecast future demand based on historical data and other relevant variables.
- Time Series Analysis: Time series analysis employs models to predict demand trends and seasonality. By analysing historical demand data over specific time periods, businesses can identify recurring patterns and seasonal variations. This allows for proactive buffer adjustments and order policy changes, ensuring inventory levels are optimised to meet anticipated demand fluctuations.
- Machine Learning Models: Implementing machine learning algorithms, such as regression, decision trees, and neural networks, significantly improves the accuracy of demand forecasts. These models can process vast amounts of data and detect complex patterns that traditional statistical methods might miss. By continuously learning from new data, machine learning models provide more reliable and nuanced forecasts, enabling better alignment of inventory with actual market conditions.
- Scenario Planning: Scenario planning involves creating multiple demand scenarios to simulate different market conditions and their impact on inventory levels and supply chain performance. By exploring various “what-if” scenarios, businesses can assess potential risks and opportunities, preparing them to respond effectively to unexpected changes in demand. This strategic foresight helps in making informed decisions about buffer sizing, order policies, and overall supply chain management.
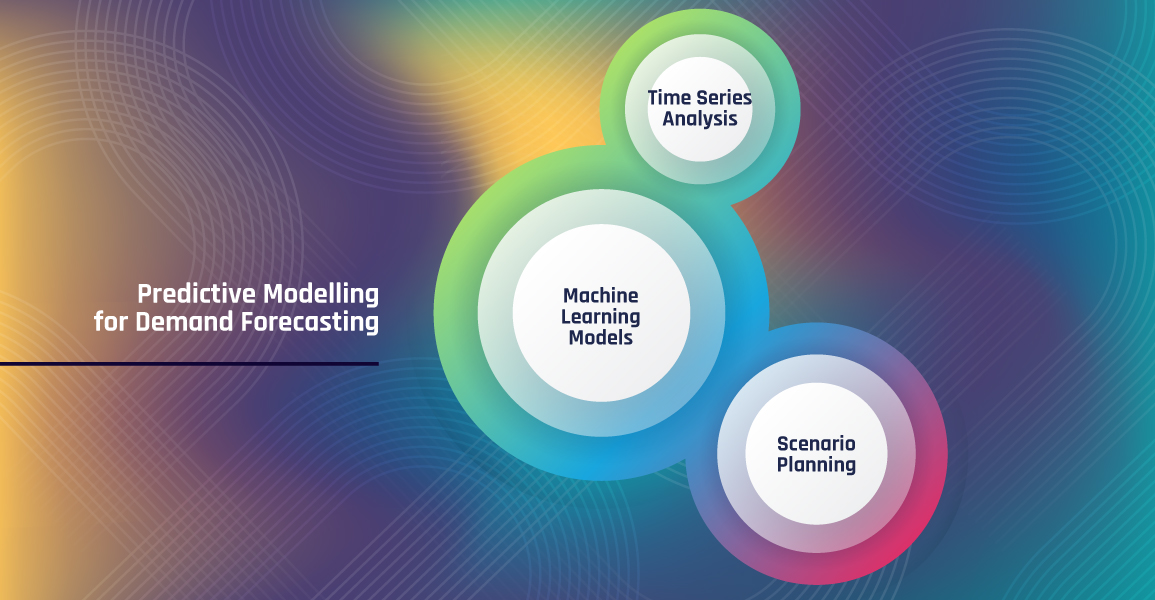
Demand Sensing Technologies: Enhancing Real-Time Responsiveness
The Concept of Demand Sensing
Demand sensing refers to the process of capturing and analysing real-time demand signals to make informed supply chain decisions. It bridges the gap between demand forecasting and actual demand, enabling more accurate and timely responses.
- Point-of-Sale Data: Leveraging point-of-sale (POS) data provides real-time insights into consumer purchasing behaviour. By analysing sales transactions as they occur, businesses can detect changes in demand patterns immediately. This timely information allows for rapid adjustments to supply chain parameters, such as inventory levels and replenishment orders, ensuring that stock is aligned with current consumer needs.
- Social Media and Market Trends: Monitoring social media trends and market indicators is another crucial aspect of demand sensing. Social media platforms and market trend analyses can reveal shifts in consumer preferences and emerging demand patterns. By tracking mentions, sentiments, and trending topics, businesses can gain early insights into what products are gaining popularity or falling out of favour, allowing them to adjust their supply chain strategies accordingly.
- Sensor Data: Utilising Internet of Things (IoT) sensors enhances demand sensing by providing real-time data on inventory levels, product movements, and environmental conditions. These sensors offer a comprehensive, up-to-the-minute view of the supply chain, enabling businesses to respond quickly to changes. For instance, if sensor data indicates that a product is running low in a specific location, immediate replenishment can be initiated to prevent stockouts.
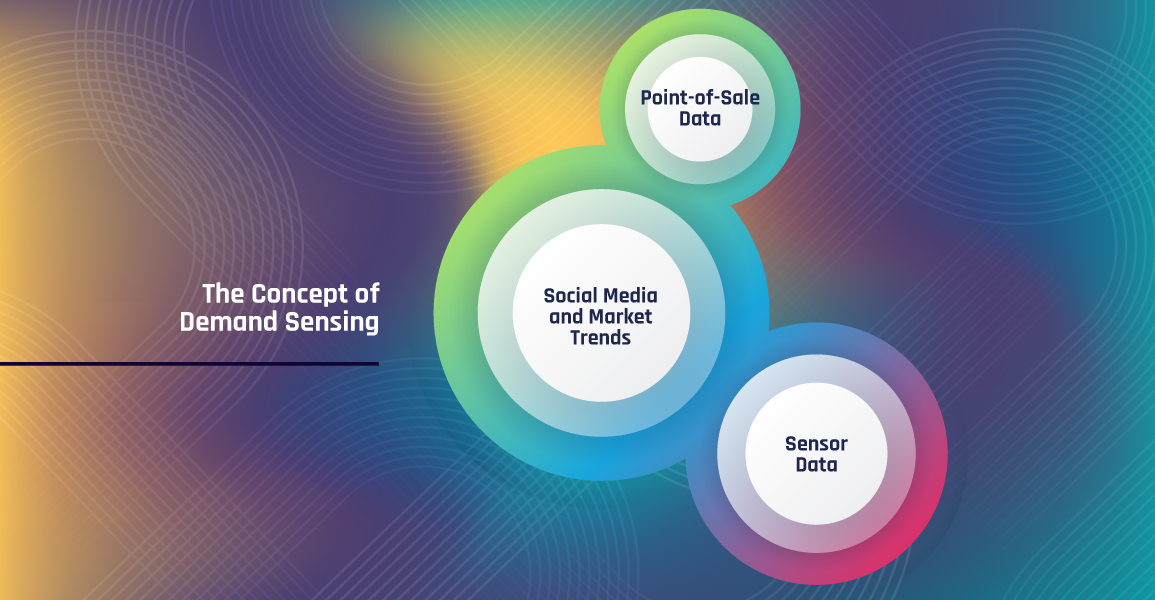
Implementing Demand Sensing in DDMRP
Integrating demand sensing technologies with DDMRP can significantly enhance the responsiveness and accuracy of demand driven planning.
- Real-Time Data Integration: Incorporating real-time demand signals into DDMRP systems is crucial for continuously adjusting buffer sizes, order policies, and lead times. By leveraging data from various sources, such as point-of-sale systems and IoT sensors, businesses can ensure that their inventory levels are always aligned with current demand. This dynamic adjustment helps in preventing stockouts and reducing excess inventory, leading to more efficient supply chain operations.
- Advanced Analytics Platforms: Using advanced analytics platforms to process and analyse demand signals provides actionable insights for supply chain optimization. These platforms can employ sophisticated algorithms and machine learning models to interpret vast amounts of data quickly, identifying trends and predicting future demand with high accuracy. The insights gained from these analytics allow businesses to make informed decisions about inventory management, ensuring that they are always prepared to meet customer needs.
- Collaborative Networks: Establishing collaborative networks with suppliers, distributors, and retailers is essential for sharing demand data and coordinating responses to market changes. By fostering open communication and data sharing, all stakeholders can stay informed about real-time demand shifts and work together to adjust their supply chain strategies accordingly. This collaborative approach enhances the overall agility and resilience of the supply chain, ensuring that it can respond effectively to both expected and unexpected changes in demand.
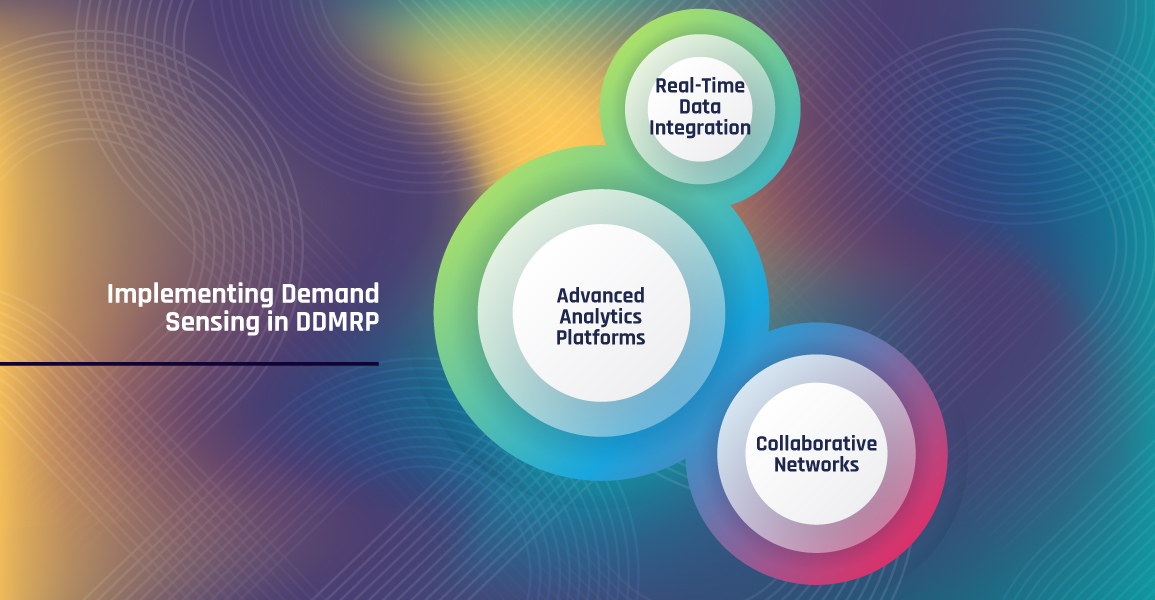
Conclusion
Dynamic Demand Management within the framework of DDMRP offers a powerful approach to optimising supply chains in the face of fluctuating market conditions. By continuously adjusting buffer sizes, order policies, and lead times based on real-time data and predictive insights, organisations can achieve greater agility, resilience, and efficiency. The integration of data analytics, predictive modelling, and demand sensing technologies further enhances the effectiveness of DDMRP, enabling supply chains to respond swiftly and accurately to changing demand patterns.
Explore innovative strategies for dynamically adjusting DDMRP parameters to effectively respond to fluctuating market conditions and demand signals.
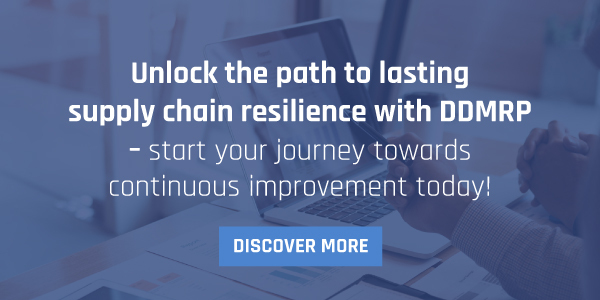