In the realm of inventory management, a paradigm shift has occurred. The traditional “push” approach, characterised by forecasts driving production and inventory, often led to mismatches between supply and demand. This mismatch resulted in overstocking, stockouts, and inefficient resource allocation. Enter Demand-Driven Material Requirements Planning (DDMRP), a methodology designed to align inventory with actual market demand. At its core, DDMRP relies on strategic decoupling points, buffers, and dynamic adjustments to create a responsive supply chain.
However, DDMRP‘s success hinges on accurate demand forecasting and agile inventory optimization. This is where the transformative power of Artificial Intelligence (AI) and Machine Learning (ML) comes into play. These technologies, with their ability to analyse vast amounts of data, identify patterns, and make predictions, are revolutionising how DDMRP is implemented and executed.
The Synergy of AI, ML, and DDMRP: A Detailed Exploration
Elevated Demand Forecasting: The Power of AI and ML in DDMRP
A fundamental weakness of traditional forecasting models lies in their dependence on historical sales data and their struggle to adapt to sudden market shifts. This can lead to inaccurate predictions, causing stock outs or excess inventory. AI and ML algorithms, however, have revolutionised demand forecasting within DDMRP.
These algorithms go beyond historical data, weaving a rich tapestry of information to create a more accurate picture of future demand. They incorporate:
- Market Trends: By analysing economic indicators, competitor actions, and industry reports, AI and ML can identify emerging trends and anticipate shifts in consumer preferences.
- Seasonality: Fluctuations in demand caused by holidays, weather patterns, or cultural events are often predictable. AI and ML algorithms can recognize these patterns and factor them into demand forecasts.
- Promotions: The impact of marketing activities, such as discounts or advertising campaigns, can significantly influence demand. AI and ML can quantify these impacts, allowing for more accurate predictions.
- Social Media Sentiment: Customer conversations, reviews, and opinions shared on social media platforms offer valuable insights into product popularity and potential demand shifts. AI and ML can analyse this sentiment data to refine forecasts.
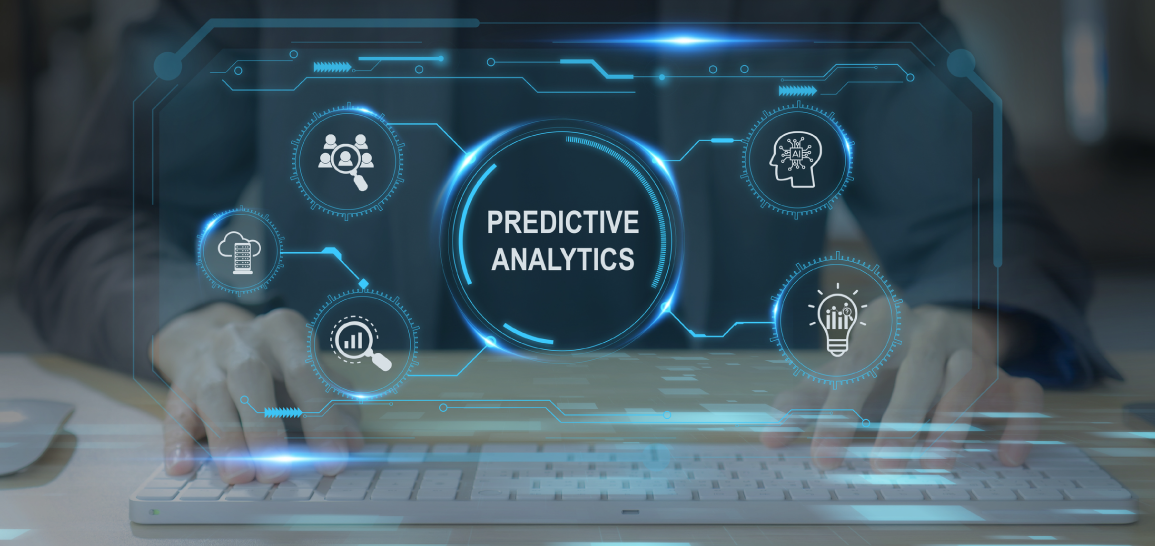
By considering this diverse range of data sources, AI and ML algorithms can generate demand forecasts that are significantly more accurate and reliable than traditional methods. These enhanced forecasts enable:
- Precise Buffer Sizing: Buffers can be set at optimal levels, ensuring adequate stock to meet demand while minimising excess inventory.
- Optimised Inventory Allocation: Inventory can be strategically distributed across locations to maximise availability and minimise transportation costs.
- Proactive Demand Adjustments: Businesses can anticipate fluctuations in demand and proactively adjust production, procurement, and inventory levels to avoid disruptions and maintain customer satisfaction.
Real-Time Inventory Optimization: AI-Powered Agility in DDMRP
A cornerstone of Demand-Driven Material Requirements Planning (DDMRP) is the use of strategic buffers. These buffers act as safety nets, absorbing shocks caused by unexpected fluctuations in demand. However, static buffers can become liabilities in a dynamic market. If buffers are too large, excess inventory ties up capital and increases holding costs. If buffers are too small, stockouts can occur, leading to lost sales and dissatisfied customers.
The solution lies in real-time inventory optimization. AI-powered analytics provide the agility needed to dynamically adjust buffers in response to changing demand signals. Here’s how it works:
- Real-Time Monitoring: AI-powered systems continuously monitor inventory levels across the supply chain. This involves tracking not only stock on hand but also incoming orders, lead times, and production schedules.
- Demand Signal Analysis: AI algorithms analyse a wide array of demand signals, including:
-
- Point-of-Sale Data: Real-time sales data provide immediate feedback on customer demand.
- Market Intelligence: AI can gather data on competitor actions, economic indicators, and industry trends to anticipate shifts in demand.
- Social Media Sentiment: Monitoring social media conversations can reveal emerging trends and changes in customer preferences.
- External Factors: Weather patterns, holidays, and special events can all impact demand. AI can factor these variables into its analysis.
- Dynamic Buffer Adjustments: Based on the analysis of real-time data and demand signals, AI algorithms can recommend adjustments to buffer levels. These adjustments can be made automatically or with human oversight, depending on the level of automation desired.
- Optimal Inventory Alignment: The goal is to ensure that inventory levels remain closely aligned with actual demand. By dynamically adjusting buffers, AI can minimise excess stock while preventing stockouts. This results in:
-
- Reduced Inventory Costs: Less capital tied up in excess inventory and lower holding costs.
- Improved Cash Flow: Faster inventory turnover and reduced working capital requirements.
- Minimised Stockouts: Fewer lost sales and improved customer satisfaction.
- Enhanced Supply Chain Efficiency: A more responsive and agile supply chain that can quickly adapt to changing market conditions.
Real-time inventory optimization through AI is a game-changer for DDMRP. It enables businesses to maintain optimal inventory levels, reduce costs, and improve customer service, all while navigating the complexities of a dynamic marketplace.
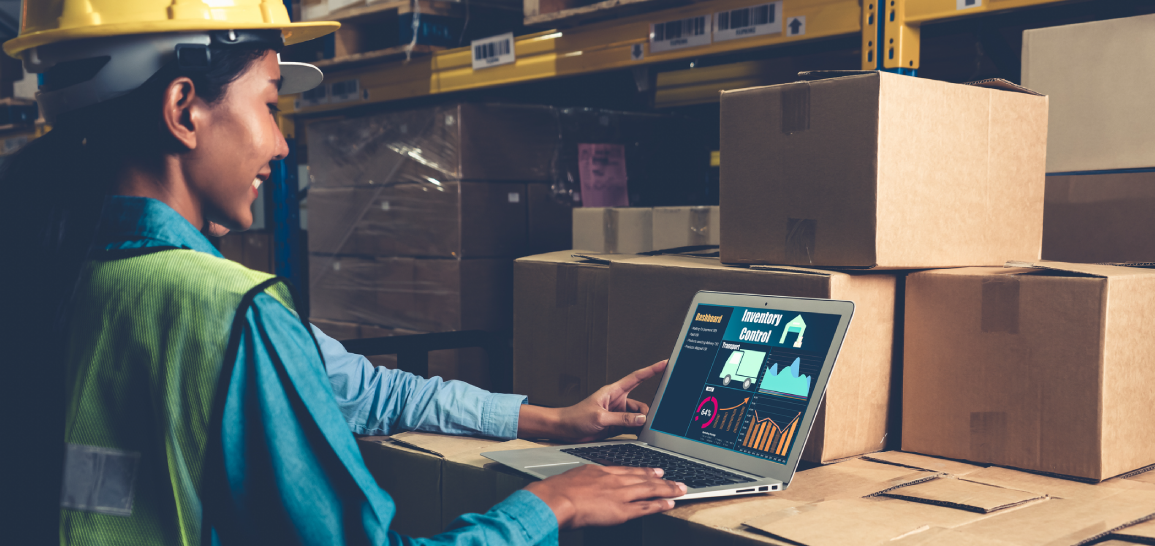
Enhanced Decision-Making: Empowering DDMRP with AI-Driven Insights
Demand-Driven Material Requirements Planning (DDMRP) is not just a methodology; it’s a complex web of interconnected decisions. Each decision, from setting buffer levels to adjusting production schedules, can significantly impact the supply chain’s overall performance. The challenge lies in making the right decisions in a timely manner, especially in the face of constantly changing market conditions and demand fluctuations.
Traditional decision-making in DDMRP often relies on human judgement, experience, and limited data analysis. While valuable, these approaches can be time-consuming, prone to bias, and limited in their ability to consider all relevant factors. This is where AI-powered decision support tools step in to revolutionise the process.
AI-Powered Decision Support: A Game-Changer for DDMRP
- Data-Driven Insights: AI algorithms can analyse vast amounts of data from various sources, including historical sales data, market trends, supplier performance, and even external factors like weather patterns or social media sentiment. This comprehensive analysis provides decision-makers with a holistic view of the supply chain, revealing hidden patterns, correlations, and potential risks.
- Scenario Simulation: AI-powered tools can simulate different scenarios, allowing decision-makers to test the impact of various choices before implementing them. For example, they can simulate the effects of different buffer levels, order quantities, or production schedules on inventory levels, costs, and customer service. This “what-if” analysis enables informed decision-making based on data-driven predictions.
- Actionable Recommendations: AI doesn’t just provide data; it transforms data into actionable insights. AI-powered decision support tools can recommend specific actions based on their analysis, such as adjusting buffer levels, expediting orders, or rerouting shipments. These recommendations are tailored to the specific situation, taking into account the current state of the supply chain and the desired outcomes.
By leveraging AI-powered decision support tools, businesses can:
- Optimise Inventory Flow: Ensure the right products are in the right place at the right time, minimising excess inventory and preventing stockouts.
- Enhance Customer Service: Improve order fulfilment rates, reduce lead times, and provide more reliable delivery dates.
- Increase Agility: Respond quickly to changing market conditions and unexpected events.
- Gain Competitive Advantage: Make better decisions faster than competitors, leading to improved market share and profitability.
AI-powered decision support tools are not meant to replace human judgement. Instead, they are designed to augment it, providing decision-makers with the information and insights they need to make the best possible choices for their supply chains. In the complex and dynamic world of DDMRP, AI can be a valuable ally, helping businesses navigate uncertainty and achieve optimal performance.
Automation of Routine Tasks: Empowering Efficiency in DDMRP
Inventory management is often bogged down by a myriad of repetitive, mundane tasks. Data collection, cleaning, analysis, and reporting are essential for effective decision-making, yet they consume valuable time and are susceptible to human error. This is where the automation capabilities of AI and ML shine, revolutionising how these tasks are handled within DDMRP.
- Data Collection and Cleaning: AI-powered tools can automate the collection of data from various sources, including ERP systems, point-of-sale terminals, warehouse management systems, and even external data providers. This eliminates the need for manual data entry, reducing the risk of errors and ensuring data consistency. Additionally, AI algorithms can identify and correct inconsistencies, errors, and missing values in the data, ensuring its accuracy and reliability.
- Data Analysis: Analysing vast amounts of inventory data can be a daunting task for human analysts. AI and ML algorithms, however, can process this data with incredible speed and accuracy. They can identify patterns, trends, and correlations that might not be obvious to the human eye. This analysis provides valuable insights into demand patterns, inventory turnover, and potential supply chain bottlenecks.
- Reporting: Generating reports on inventory levels, demand forecasts, and performance metrics is often a time-consuming process. AI can automate the generation of customised reports, delivering them directly to relevant stakeholders. These reports can be tailored to specific needs, providing insights into key performance indicators and highlighting areas for improvement.
- Exception Handling: While AI and ML can handle routine tasks, they are not infallible. Exceptions and anomalies still need to be addressed by human experts. However, by automating routine tasks, AI frees up these experts to focus their attention on exception handling, ensuring that critical issues are addressed promptly and effectively.
- Continuous Improvement: AI and ML models can continuously learn and improve over time. By analysing data and feedback, they can refine their algorithms, leading to more accurate predictions, better decision-making, and continuous improvement in inventory management processes.
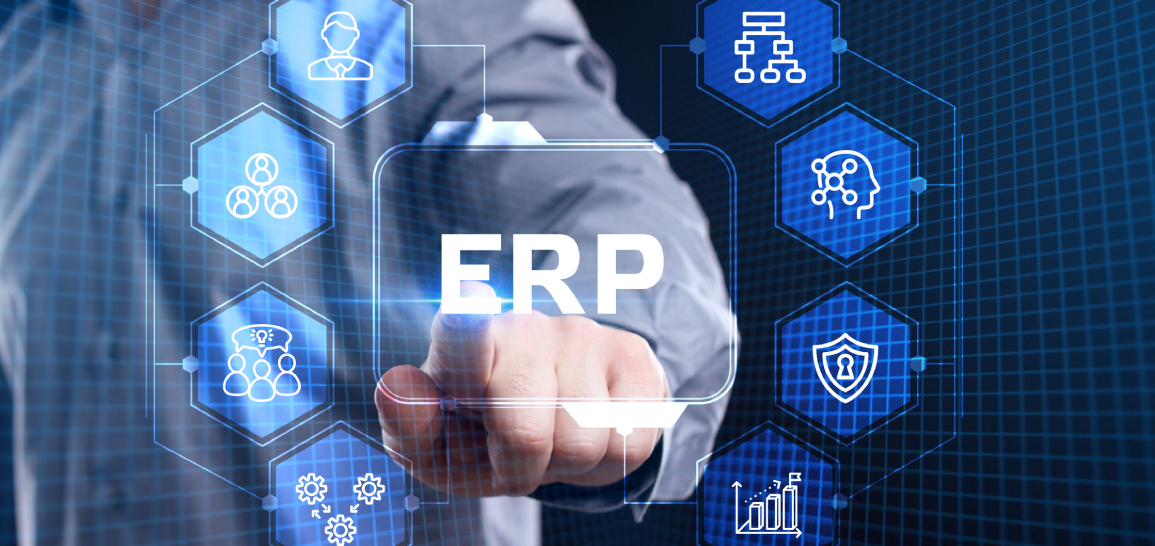
The benefits of automation in DDMRP are numerous:
- Improved Efficiency: By automating repetitive tasks, AI and ML free up valuable human resources, allowing them to focus on strategic activities that require creativity, critical thinking, and problem-solving skills.
- Increased Accuracy: Automation reduces the risk of human error in data processing, ensuring that decisions are based on accurate and reliable information.
- Faster Decision-Making: By providing real-time insights and recommendations, AI-powered tools enable faster and more informed decision-making.
- Cost Savings: Automation can reduce labour costs associated with manual data processing and improve inventory management efficiency, leading to cost savings in the long run.
- Enhanced Scalability: Automated systems can easily scale to handle larger volumes of data and more complex supply chains, making them ideal for growing businesses.
By embracing automation, businesses can unlock the full potential of DDMRP, achieving greater efficiency, accuracy, and responsiveness in their inventory management processes.
The Future of AI and ML in DDMRP: A Glimpse into the Next Frontier
The evolution of AI and ML is far from over, and their impact on Demand-Driven Material Requirements Planning (DDMRP) is poised to expand exponentially. We stand on the brink of groundbreaking advancements that will redefine how businesses manage inventory and supply chains.
- Explainable AI (XAI): A significant hurdle in adopting AI has been the “black box” nature of many algorithms. Explainable AI aims to address this by making AI models transparent and understandable. In the context of DDMRP, XAI would enable planners to understand the reasoning behind AI-generated recommendations, increasing trust and facilitating better decision-making. This transparency fosters collaboration between humans and AI, where human expertise can complement AI’s analytical capabilities.
- Reinforcement Learning (RL): Traditional AI models rely on pre-existing data for training. RL, however, takes a different approach. It allows AI agents to learn through trial and error, interacting with their environment and receiving feedback in the form of rewards or penalties. In DDMRP, RL could be used to optimise buffer levels, order quantities, and other parameters in real-time. By continuously learning from its actions and adapting to changing conditions, RL-powered DDMRP systems could achieve unprecedented levels of responsiveness and efficiency.
- Integration with the Internet of Things (IoT): The proliferation of IoT devices presents a wealth of opportunities for DDMRP. Sensors embedded in products, warehouses, and transportation vehicles can provide real-time data on inventory levels, product movements, storage conditions, and even potential disruptions. By integrating this data into DDMRP systems, AI and ML algorithms can create a digital twin of the supply chain, enabling proactive decision-making and risk mitigation.
- Collaboration with Blockchain: Blockchain technology offers a secure, transparent, and immutable way to track the movement of goods throughout the supply chain. By combining blockchain with AI and ML, businesses can create a tamper-proof record of inventory transactions, enhancing traceability and accountability. This could be particularly valuable for industries with complex supply chains, such as pharmaceuticals or luxury goods, where counterfeit products are a concern.
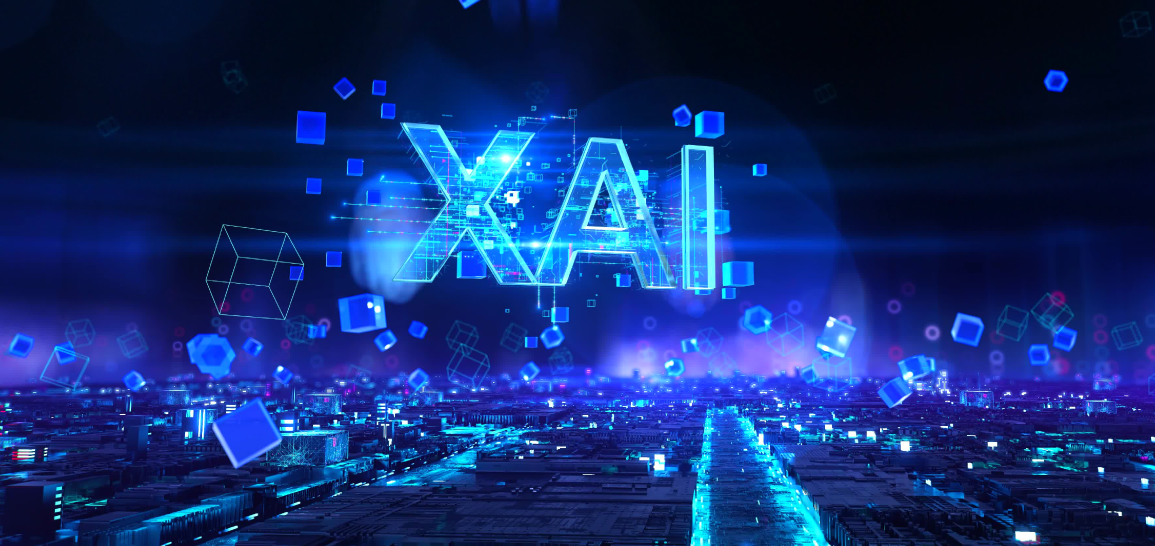
In addition to these advancements, we can expect further integration of AI and ML with other emerging technologies like natural language processing (NLP) and computer vision. NLP could enable DDMRP systems to understand and respond to human language, while computer vision could be used for inventory tracking and quality control.
The future of AI and ML in DDMRP is bright. These technologies hold the key to unlocking a new era of intelligent, adaptive, and resilient supply chains. By embracing these advancements, businesses can position themselves at the forefront of innovation, gaining a competitive edge in the ever-evolving marketplace.
Conclusion
The journey towards intelligent inventory management has just begun, and the possibilities are endless. As we move forward, the continued collaboration between human expertise and AI capabilities will be essential in shaping a future where supply chains are not just efficient but also intelligent, adaptable, and sustainable. The dawn of intelligent inventory management is here, and it promises to revolutionise the way businesses operate and compete in the global marketplace.
Discover how AI can revolutionise your DDMRP-enabled inventory management processes. Get in touch today!
